What is A/B Testing: Know the Secrets of Data-Driven Decision-Making
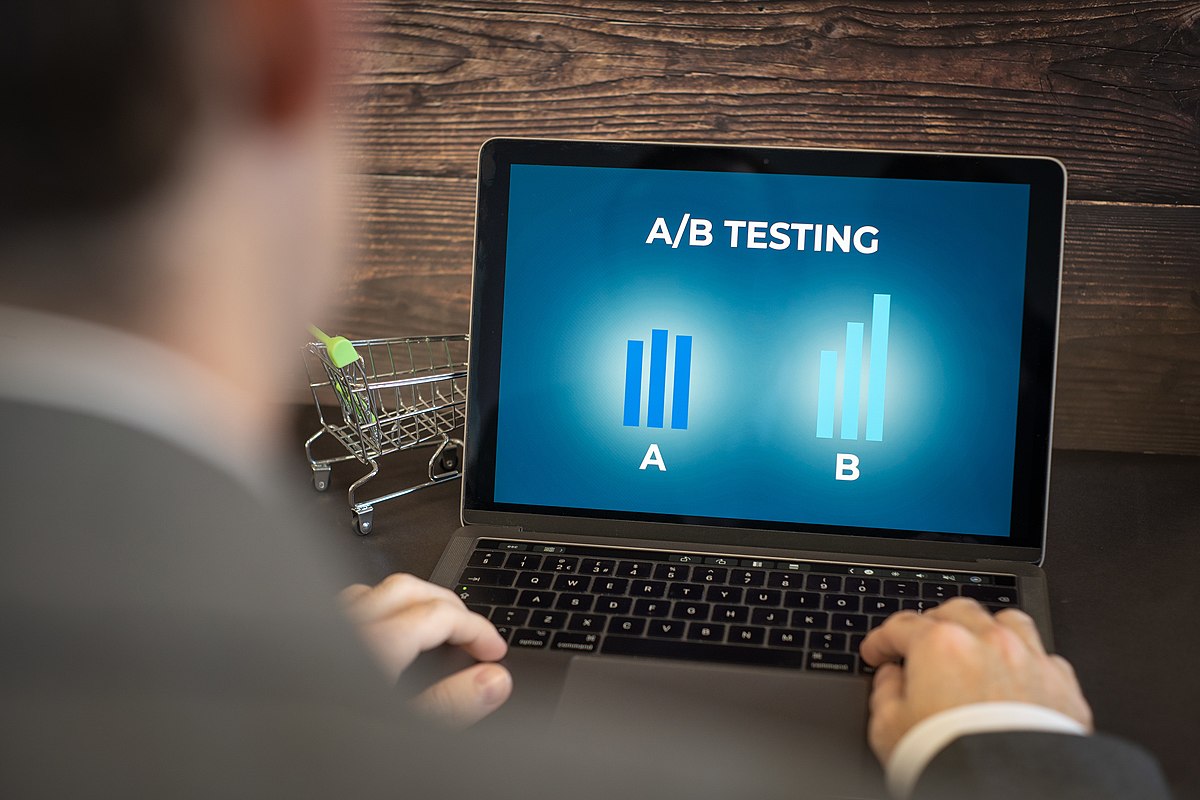
Introduction
In the ever-evolving landscape of digital marketing and web development, A/B testing stands out as a crucial tool for optimizing strategies and improving user experiences. This methodological approach allows businesses to make informed decisions based on concrete data rather than assumptions. This article delves into the depths of A/B testing, unraveling its intricacies and showcasing its transformative power in refining online performance.
I. Understanding A/B Testing
A. Definition and Basics
A/B testing, also known as split testing, is a method wherein two versions of a webpage or app are compared to determine which one performs better. It involves dividing the audience into two groups—A and B—and exposing each group to a different version. The performance metrics are then analyzed to identify the version that yields superior results.
B. The Science Behind A/B Testing
- Hypothesis Formulation
Before initiating an A/B test, a clear hypothesis must be established. This involves identifying a specific element on the webpage or app that you want to test, such as a headline, call-to-action button, or color scheme. The hypothesis should articulate the expected impact on user behavior.
- Randomization
Randomly assigning users to either the control group (A) or the variant group (B) is crucial to ensuring a representative sample. This minimizes the risk of skewed results due to external factors and ensures that the comparison accurately reflects user preferences.
II. Implementing A/B Testing
A. Selecting Variables for Testing
- Landing Page Elements
Landing pages play a pivotal role in user engagement. Testing variables like headline copy, images, and overall layout can provide valuable insights into what resonates with your audience.
- Call-to-Action Buttons
The design, color, and placement of call-to-action buttons significantly influence user conversions. A/B testing different variations can pinpoint the most effective configuration.
B. Tools and Platforms
- Google Optimize
Google Optimize is a user-friendly tool that seamlessly integrates with Google Analytics. It allows for easy creation and execution of A/B tests, providing in-depth reports to facilitate data-driven decisions.
- Optimizely
Optimizely is a robust experimentation platform that enables businesses to test various elements of their digital properties. With its advanced features, Optimizely caters to both small-scale and enterprise-level A/B testing.
III. Analyzing A/B Test Results
A. Key Metrics to Evaluate
- Conversion Rate
The conversion rate is a fundamental metric indicating the percentage of users who complete the desired action. Whether it’s making a purchase, signing up, or downloading, a higher conversion rate is generally indicative of a more effective design.
- Click-Through Rate (CTR)
CTR measures the ratio of users who click on a specific element, such as a link or button, to the number of total users. A higher CTR suggests that the tested variable is resonating positively with the audience.
B. Statistical Significance
Interpreting A/B test results requires a grasp of statistical significance. This ensures that the observed differences between the control and variant groups are not due to random chance. Tools like the chi-squared test and t-test help determine the reliability of the results.
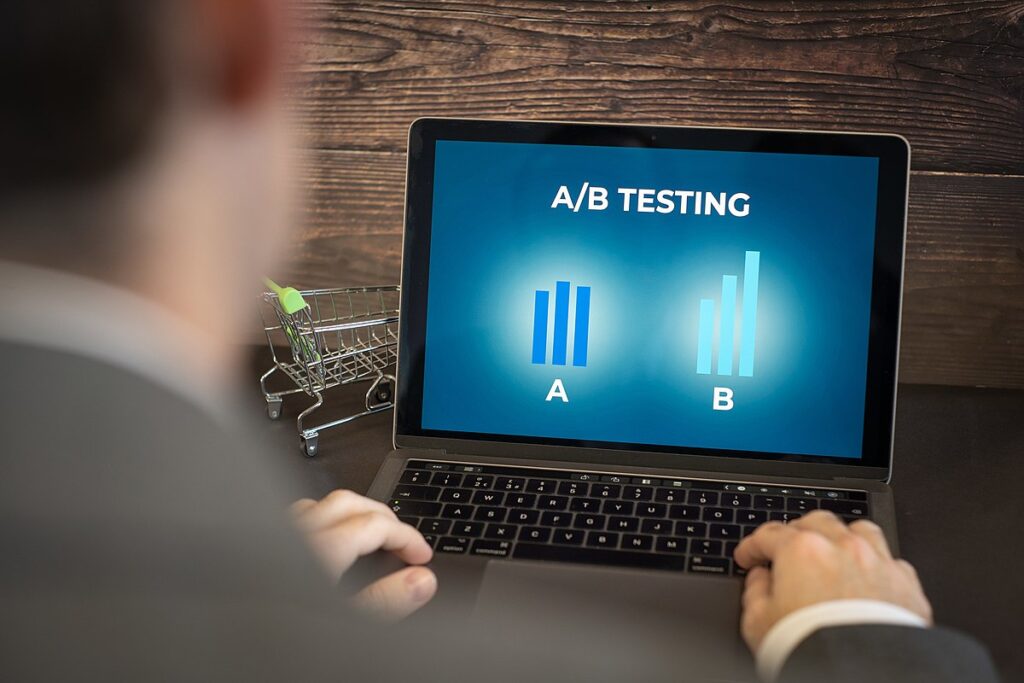
IV. Best Practices for A/B Testing
A. Sequential Testing
Instead of waiting for a predetermined duration, consider implementing sequential testing. This approach allows you to monitor results in real time and halt the test once statistical significance is reached, optimizing efficiency.
B. Continuous Iteration
A/B testing is not a one-time affair. Continuous iteration based on insights gained from multiple tests is essential for staying ahead of changing user preferences and market dynamics.
V. Overcoming Common A/B Testing Challenges
A. Sample Size Issues
Small sample sizes can lead to inconclusive or unreliable results. Ensuring an adequate number of participants is essential for drawing accurate conclusions.
B. External Variables
External factors, such as seasonality or marketing campaigns, can impact A/B test results. Careful consideration and control for these variables are necessary for accurate interpretation.
Conclusion: In conclusion, A/B testing is a powerful tool that empowers businesses to make data-driven decisions, optimize user experiences, and maximize conversions. By understanding the basics, implementing tests effectively, and interpreting results accurately, organizations can unlock the full potential of A/B testing. As the digital landscape continues to evolve, mastering this methodology will be a cornerstone for success in the competitive online arena.
- Unlocking the Secrets: How to Become a Successful YouTuber - March 17, 2024
- Unlocking the Power of Home Loan Calculators: A Comprehensive Guide - March 9, 2024
- Understanding Dementia: A Comprehensive Guide - March 7, 2024